Beyerol 17-cinnamate
* Please be kindly noted products are not for therapeutic use. We do not sell to patients.

Category | Others |
Catalog number | BBF-05474 |
CAS | 59219-79-3 |
Molecular Weight | 450.61 |
Molecular Formula | C29H38O4 |
Online Inquiry
Description
It is produced by the strain of Beyeria (euphorbiaceae) leschenaultii var. drummondii.
Specification
Synonyms | 17-Norkaur-15-ene-3,18-diol, 13-[[(1-oxo-3-phenyl-2-propen-1-yl)oxy]methyl]-, (3alpha,4alpha,8beta,13beta)-; 17-Norkaur-15-ene-3,18-diol, 13-[[(1-oxo-3-phenyl-2-propen-1-yl)oxy]methyl]-, (3α,4α,8β,13β)-; beyerol 17-monocinnamate; (8β,13R)-13-Cinnamoyloxymethyl-17-norkaur-15-ene-3β,18-diol; ((3R,4R,4aS,6aR,9R,11aS,11bS)-3-hydroxy-4-(hydroxymethyl)-4,11b-dimethyl-2,3,4,4a,5,6,10,11,11a,11b-decahydro-6a,9-methanocyclohepta[a]naphthalen-9(1H)-yl)methyl cinnamate |
IUPAC Name | [(1R,4S,5R,6S,9S,10S,13R)-6-hydroxy-5-(hydroxymethyl)-5,9-dimethyl-13-tetracyclo[11.2.1.01,10.04,9]hexadec-14-enyl]methyl (E)-3-phenylprop-2-enoate |
Canonical SMILES | CC12CCC(C(C1CCC34C2CCC(C3)(C=C4)COC(=O)C=CC5=CC=CC=C5)(C)CO)O |
InChI | InChI=1S/C29H38O4/c1-26-13-12-24(31)27(2,19-30)22(26)11-15-29-17-16-28(18-29,14-10-23(26)29)20-33-25(32)9-8-21-6-4-3-5-7-21/h3-9,16-17,22-24,30-31H,10-15,18-20H2,1-2H3/b9-8+/t22-,23-,24-,26+,27-,28-,29-/m0/s1 |
InChI Key | TVPQCJPVUOWJET-LVTIAXTASA-N |
Reference Reading
1. Artificial Intelligence in Oncological Hybrid Imaging
Benedikt Feuerecker, Maurice M Heimer, Thomas Geyer, Matthias P Fabritius, Sijing Gu, Balthasar Schachtner, Leonie Beyer, Jens Ricke, Sergios Gatidis, Michael Ingrisch, Clemens C Cyran Rofo. 2023 Feb;195(2):105-114. doi: 10.1055/a-1909-7013. Epub 2022 Sep 28.
Background: Artificial intelligence (AI) applications have become increasingly relevant across a broad spectrum of settings in medical imaging. Due to the large amount of imaging data that is generated in oncological hybrid imaging, AI applications are desirable for lesion detection and characterization in primary staging, therapy monitoring, and recurrence detection. Given the rapid developments in machine learning (ML) and deep learning (DL) methods, the role of AI will have significant impact on the imaging workflow and will eventually improve clinical decision making and outcomes. Methods and results: The first part of this narrative review discusses current research with an introduction to artificial intelligence in oncological hybrid imaging and key concepts in data science. The second part reviews relevant examples with a focus on applications in oncology as well as discussion of challenges and current limitations. Conclusion: AI applications have the potential to leverage the diagnostic data stream with high efficiency and depth to facilitate automated lesion detection, characterization, and therapy monitoring to ultimately improve quality and efficiency throughout the medical imaging workflow. The goal is to generate reproducible, structured, quantitative diagnostic data for evidence-based therapy guidance in oncology. However, significant challenges remain regarding application development, benchmarking, and clinical implementation. Key points: · Hybrid imaging generates a large amount of multimodality medical imaging data with high complexity and depth.. · Advanced tools are required to enable fast and cost-efficient processing along the whole radiology value chain.. · AI applications promise to facilitate the assessment of oncological disease in hybrid imaging with high quality and efficiency for lesion detection, characterization, and response assessment. The goal is to generate reproducible, structured, quantitative diagnostic data for evidence-based oncological therapy guidance.. · Selected applications in three oncological entities (lung, prostate, and neuroendocrine tumors) demonstrate how AI algorithms may impact imaging-based tasks in hybrid imaging and potentially guide clinical decision making.. Citation format: · Feuerecker B, Heimer M, Geyer T et al. Artificial Intelligence in Oncological Hybrid Imaging. Fortschr Röntgenstr 2023; 195: 105 - 114.
2. Update on CSF Biomarkers in Parkinson's Disease
Eun Hae Kwon, Sabrina Tennagels, Ralf Gold, Klaus Gerwert, Léon Beyer, Lars Tönges Biomolecules. 2022 Feb 18;12(2):329. doi: 10.3390/biom12020329.
Progress in developing disease-modifying therapies in Parkinson's disease (PD) can only be achieved through reliable objective markers that help to identify subjects at risk. This includes an early and accurate diagnosis as well as continuous monitoring of disease progression and therapy response. Although PD diagnosis still relies mainly on clinical features, encouragingly, advances in biomarker discovery have been made. The cerebrospinal fluid (CSF) is a biofluid of particular interest to study biomarkers since it is closest to the brain structures and therefore could serve as an ideal source to reflect ongoing pathologic processes. According to the key pathophysiological mechanisms, the CSF status of α-synuclein species, markers of amyloid and tau pathology, neurofilament light chain, lysosomal enzymes and markers of neuroinflammation provide promising preliminary results as candidate biomarkers. Untargeted approaches in the field of metabolomics provide insights into novel and interconnected biological pathways. Markers based on genetic forms of PD can contribute to identifying subgroups suitable for gene-targeted treatment strategies that might also be transferable to sporadic PD. Further validation analyses in large PD cohort studies will identify the CSF biomarker or biomarker combinations with the best value for clinical and research purposes.
3. Tau deposition patterns are associated with functional connectivity in primary tauopathies
Nicolai Franzmeier, Matthias Brendel, Leonie Beyer, et al. Nat Commun. 2022 Mar 15;13(1):1362. doi: 10.1038/s41467-022-28896-3.
Tau pathology is the main driver of neuronal dysfunction in 4-repeat tauopathies, including cortico-basal degeneration and progressive supranuclear palsy. Tau is assumed to spread prion-like across connected neurons, but the mechanisms of tau propagation are largely elusive in 4-repeat tauopathies, characterized not only by neuronal but also by astroglial and oligodendroglial tau accumulation. Here, we assess whether connectivity is associated with 4R-tau deposition patterns by combining resting-state fMRI connectomics with both 2nd generation 18F-PI-2620 tau-PET in 46 patients with clinically diagnosed 4-repeat tauopathies and post-mortem cell-type-specific regional tau assessments from two independent progressive supranuclear palsy patient samples (n = 97 and n = 96). We find that inter-regional connectivity is associated with higher inter-regional correlation of both tau-PET and post-mortem tau levels in 4-repeat tauopathies. In regional cell-type specific post-mortem tau assessments, this association is stronger for neuronal than for astroglial or oligodendroglial tau, suggesting that connectivity is primarily associated with neuronal tau accumulation. Using tau-PET we find further that patient-level tau patterns are associated with the connectivity of subcortical tau epicenters. Together, the current study provides combined in vivo tau-PET and histopathological evidence that brain connectivity is associated with tau deposition patterns in 4-repeat tauopathies.
Recommended Products
BBF-05762 | Cyclosporin B | Inquiry |
BBF-03915 | Clavulanate Potassium (1:1 mixture with silicon dioxide) | Inquiry |
BBF-03884 | Formononetin | Inquiry |
BBF-00968 | Homoalanosine | Inquiry |
BBF-02582 | Polyporenic acid C | Inquiry |
BBF-04609 | 1,1-Dimethylbiguanide hydrochloride | Inquiry |
Bio Calculators
* Our calculator is based on the following equation:
Concentration (start) x Volume (start) = Concentration (final) x Volume (final)
It is commonly abbreviated as: C1V1 = C2V2
* Total Molecular Weight:
g/mol
Tip: Chemical formula is case sensitive. C22H30N4O √ c22h30n40 ╳
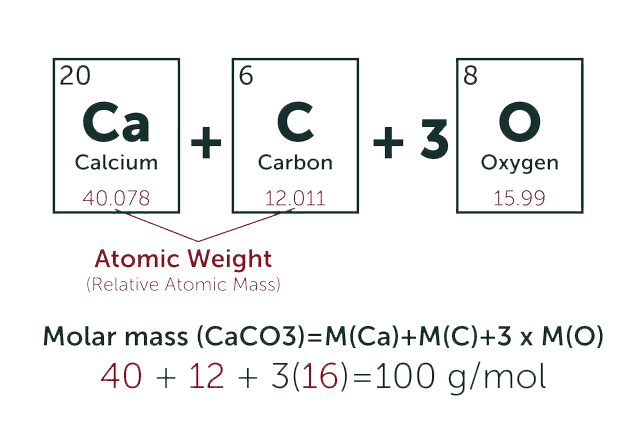